Introduction
The Big Data
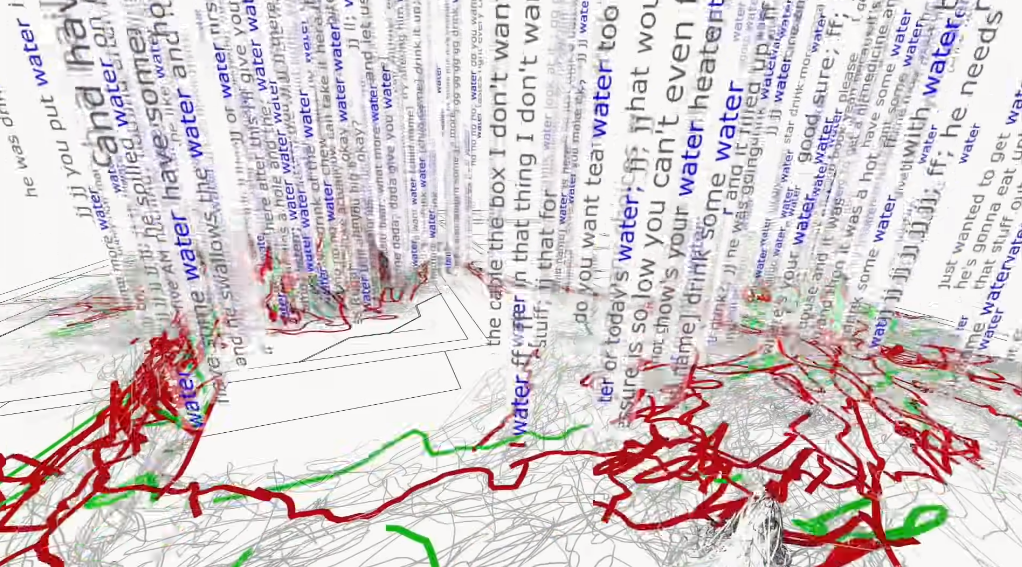
As the world becomes increasingly digitized, businesses and organizations are collecting vast amounts of data. This data has become a valuable commodity and is used to make strategic decisions, improve marketing efforts, and develop new products and services. However, with so much information being collected, it’s essential to ensure that it’s protected from cybercriminals.
Big Data Security refers to the measures taken by organizations to safeguard their sensitive information from unauthorized access, theft, or damage. The unique aspects of Big Data security include the need for real-time monitoring and analysis of large amounts of data, as well as the ability to detect anomalies quickly. In addition, Big Data security requires advanced threat intelligence capabilities that can identify potential threats before they occur.
The Growing Importance of Big Data Security
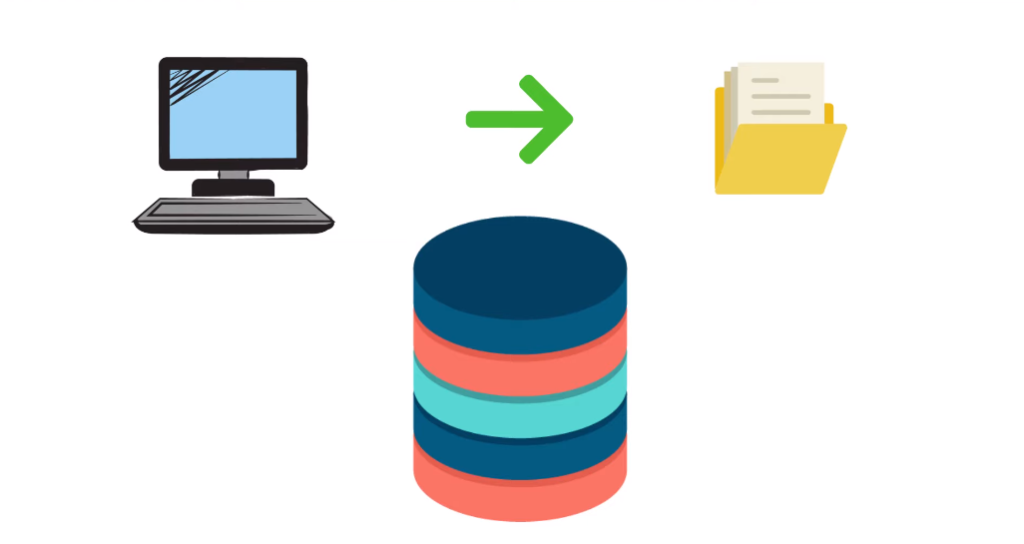
As the amount of data generated continues to accelerate, so does the need for effective and efficient big data security measures. Big data is characterized by its volume, velocity, and variety, which present unique challenges for securing it against potential threats. With the vast amount of sensitive information contained within big data sets – including financial records, personal identification details, and intellectual property – a breach could have catastrophic consequences.
Understanding Big Data
Definition and Characteristics of Big Data
Big data refers to large sets of structured, semi-structured, or unstructured data that traditional data processing tools and methods cannot efficiently handle.
The Three V’s of Big Data (Volume, Velocity, Variety)
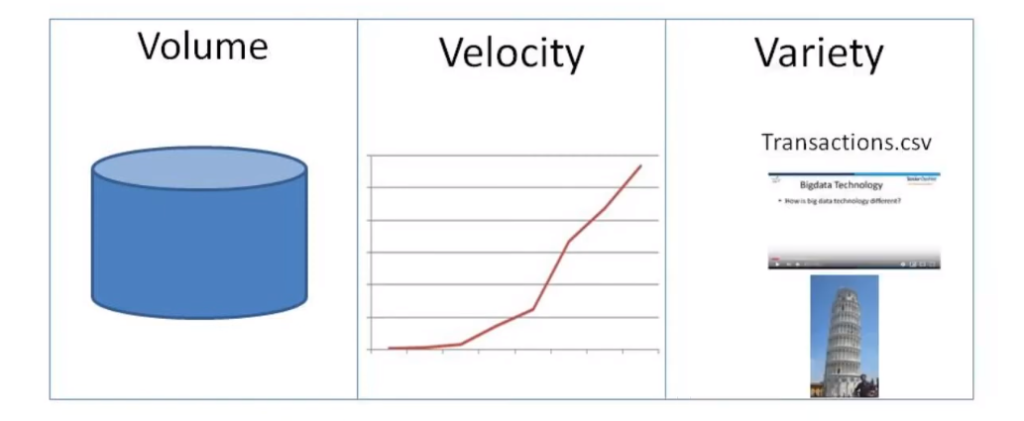
Big data is characterized by three key features, known as the three V’s: volume, velocity, and variety. The first V refers to the massive amounts of data that are generated and collected from various sources such as social media platforms, IoT devices, and other digital channels. This sheer volume of data creates significant challenges for organizations in terms of storage, processing power, and analysis.
The second V – velocity – describes the speed at which data is generated and must be processed in real time. With the increasing number of users generating vast amounts of information every second through clicks, likes, or shares on social media platforms or browsing behaviors on websites; traditional methods for processing data are no longer enough. Therefore companies need to make use of advanced technologies such as machine learning algorithms that can work with high-speed streams of information.
Lastly comes variety – this is about understanding the different types and formats of data that exist today. Data can take many forms such as structured (data stored in databases), unstructured (videos or audio), semi-structured (emails), etc., which makes it challenging to analyze them using traditional methods.
The Advantages of Harnessing Big Data
Big data is becoming an increasingly valuable resource for businesses across all industries. The ability to collect and analyze vast amounts of data enables companies to identify patterns, trends, and insights that were previously impossible to uncover. This information can be used to make informed decisions in areas such as marketing, customer service, product development, and supply chain management. Ultimately, harnessing big data gives businesses a competitive advantage by allowing them to stay ahead of the curve.
Another advantage of big data is its potential for improving cybersecurity. By analyzing large volumes of data from various sources, companies can detect anomalies and potential threats more quickly and accurately than ever before. This allows them to take proactive measures to prevent cyber attacks before they occur or minimize their impact if they do occur.
The Unique Challenges of Big Data Security
Scale and Complexity of Data
Big data is much more than just a large amount of information. It involves vast volumes of unstructured or semi-structured data that require advanced analytics to extract insights. The scale and complexity of big data pose significant challenges for businesses, particularly when it comes to security. As the volume of data grows, so does the risk of cyber threats and breaches.
Furthermore, the complexity of big data means that it is not always easy to identify what information is sensitive or confidential. Data may be stored in different locations across multiple platforms and devices, making it difficult to keep track of who has access to which parts of the dataset. This fragmentation can also make it challenging to implement effective security measures that cover all aspects of both structured and unstructured data.
Data Collection and Storage
Data is collected from various sources such as social media, sensors, customer interactions, and financial transactions. The data sets can be massive and complex, making it important to have a robust storage system in place to handle the volume. This requires a scalable infrastructure that can accommodate growth while maintaining performance.
In addition to storage, data must be secured during collection and throughout its lifecycle. Big data security is paramount due to the sensitive information it contains such as personal identification details, financial records, intellectual property rights, and confidential company information among others.
Data Variety and Heterogeneity
Data Variety and Heterogeneity are two of the most significant challenges in big data security. In today’s world, data is being generated from a wide range of sources, such as social media platforms, IoT devices, mobile apps, and more. With this influx of data comes increased complexity in managing it. The heterogeneity of big data makes it difficult for organizations to integrate and analyze all the various types of information.
Moreover, the variety and heterogeneity make it harder to ensure data quality and accuracy when processing large amounts of information. This issue can lead to incorrect conclusions being drawn from the analysis or even worse; incorrect decisions made based on those conclusions.
Data Accessibility and Sharing
Maintaining both accessibility and security when dealing with big data can be a delicate balancing act. By establishing clear protocols for managing sensitive information and working closely with trusted partners, businesses can help ensure that their valuable datasets remain secure while still allowing them to leverage the power of big data analytics.
Data Privacy in Big Data
Privacy Risks and Concerns
Privacy risks and concerns are prevalent in the world of big data security. Big data analytics can reveal sensitive information about an individual’s online activities, shopping habits, financial status, health records, and more. This information can be used without consent or knowledge to make decisions that could impact an individual’s life.
Moreover, cybercriminals are always on the lookout for ways to breach databases and steal personal information. As such, companies must ensure they have robust security measures in place to protect their customers’ data from unauthorized access or theft. The consequences of a privacy breach can be severe for both individuals and companies alike – loss of trust from customers leading to reputational damage for businesses; identity theft leading to financial losses for individuals.
Legal and Regulatory Frameworks
In the age of Big Data, data privacy has become an increasingly important issue. As companies collect more and more information on their customers, it is essential that they do so in a way that is both legally compliant and respectful of individual privacy rights. To achieve this, businesses must navigate a complex web of legal and regulatory frameworks designed to protect consumer data.
In the United States, for example, there are several laws regulating data privacy such as the Health Insurance Portability and Accountability Act (HIPAA), Children’s Online Privacy Protection Act (COPPA), and General Data Protection Regulation (GDPR) in Europe among others. While these laws differ based on jurisdiction or industry, businesses need to understand how they impact their operations to stay compliant. Failure to comply with these regulations can result in hefty fines or even lawsuits.
To ensure compliance with legal frameworks governing big data security, businesses should have a dedicated team responsible for monitoring compliance issues regularly. They should also use security systems designed specifically for handling sensitive data while providing regular training sessions for employees on best practices surrounding privacy protection.
Anonymization and Pseudonymization Techniques
Anonymization involves removing any identifying information from the data, making it impossible to link back to an individual or entity. This technique is often used when sharing data with third parties for research purposes. Pseudonymization, on the other hand, involves replacing identifiable information with a pseudonym or code. This technique allows for personalized analysis while protecting the privacy of individuals.
There are various methods of anonymization and pseudonymization, including generalization, suppression, and hashing. Generalization involves reducing the level of detail in data by grouping similar values together. Suppression refers to removing sensitive information altogether from a dataset. Hashing involves transforming data into unique codes that cannot be reversed without access to a special key.
Security Risks in Big Data
Data Breaches and Unauthorized Access
Data breaches and unauthorized access are two of the most significant risks associated with big data security. As organizations increasingly rely on big data to make critical business decisions, protecting sensitive information from cybercriminals becomes more vital than ever before.
A data breach occurs when hackers gain unauthorized access to an organization’s computer systems or networks and steal valuable information such as personal identification numbers, credit card information, or intellectual property. Such incidents can lead to devastating consequences for companies like financial losses, loss of reputation, and legal actions taken against them by affected customers/clients.
Unauthorized access is another major challenge for big data security. It occurs when an individual gains entry into a system without permission or exceeds their authorized level of access. This type of attack can be particularly severe in organizations that store sensitive data such as patient records or financial transactions.
Insider Threats and Data Misuse
This type of threat comes from within an organization, where employees or authorized personnel have access to sensitive data that can be misused intentionally or unintentionally. Insider threats can range from employees stealing confidential information for personal gain, selling it to third-party sources or competitors, or simply accidentally sharing information with unauthorized parties.
The consequences of insider threats and data misuse can be catastrophic for organizations as it not only affects their reputation but also causes financial losses. In addition, insider attacks are often difficult to detect as they come from within the organization’s trusted network.
Data Integrity and Quality Issues
Inaccurate or incomplete data can significantly impact decision-making processes, leading to costly mistakes. Ensuring data accuracy is crucial in maintaining the trust of customers and stakeholders.
One of the main reasons for poor data quality is the lack of standardization across different systems and databases. Data may be stored in various formats, making it difficult to integrate and analyze properly. Additionally, human error during data entry can also lead to inaccuracies.
Distributed Denial of Service (DDoS) Attacks
Distributed Denial of Service (DDoS) attacks are a type of cyber attack that aims to overwhelm websites, servers, or networks with traffic from multiple sources. The attackers use botnets – networks of compromised devices such as computers and IoT devices – to flood the target with traffic until it crashes or becomes inaccessible to legitimate users. DDoS attacks have become increasingly common in recent years, and their scale and complexity continue to grow.
Scalability and Performance
Managing Security in High-Volume Data Environments
One key aspect of managing security in high-volume data environments is access control. Companies must implement strict controls over who has access to sensitive information, and how it can be accessed. This includes limiting access based on job roles and responsibilities, as well as implementing multi-factor authentication protocols.
Encryption is another important tool in securing high-volume data environments. By encrypting sensitive information both at rest and in transit, companies can ensure that even if a cyber attack occurs, the attacker will not be able to read or interpret the stolen data. In addition, encryption helps protect against insider threats by making it more difficult for employees with malicious intent to steal sensitive information.
Real-Time Monitoring and Analytics
Organizations have to implement proper tools that allow for real-time analysis of security events within their systems. Real-time monitoring ensures that any suspicious activity or attempted breach is detected immediately, enabling quick response and mitigation actions before any damage is done.
To achieve real-time monitoring, organizations use advanced analytic tools that can process large volumes of data in real-time, enabling them to detect anomalies and identify potential threats accurately. These tools use machine learning algorithms to learn from the data patterns and identify unusual behavior that might indicate a threat. Real-time analytics also allows organizations to track user activity from different devices and locations, ensuring better visibility into user behavior.
Scalable Security Infrastructure
Traditional security solutions are often unable to handle the vast amount of data generated by big data applications. As a result, companies are turning to scalable security solutions that can keep up with their growing data needs.
One approach to building a scalable security infrastructure is through the use of cloud-based solutions. Cloud-based security services offer a high degree of scalability and flexibility, allowing organizations to easily add or remove resources as needed. Additionally, they can be accessed from anywhere, making them ideal for organizations that have multiple locations or remote workers.
Another approach is to implement a distributed security architecture. This involves distributing security functions across multiple nodes in a network, rather than relying on a single centralized system. By decentralizing their security infrastructure, organizations can improve performance and reduce the risk of single points of failure.
Machine Learning for Big Data Security
Leveraging Machine Learning Algorithms
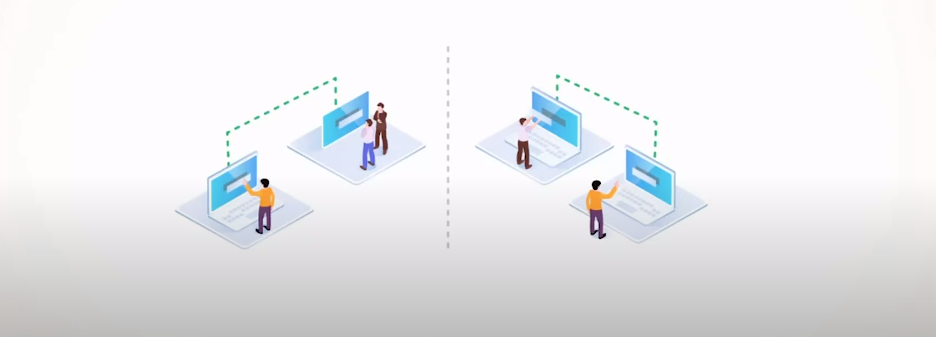
Machine learning algorithms are a powerful tool to help businesses improve their big data security. These algorithms can be used to detect patterns and anomalies in large datasets, making it easier to identify potential security threats before they become major problems. By leveraging machine learning techniques, companies can achieve greater accuracy in identifying both known and unknown threats, reducing the risk of data breaches and other security incidents.
One key advantage of using machine learning algorithms for big data security is their ability to adapt over time. As new threats emerge, these algorithms can be updated and trained on new data sets to better detect them in the future. This makes them an ideal solution for companies that need to stay ahead of constantly evolving security risks.
Detecting Anomalies and Patterns
Anomalies are deviations from the expected behavior, which could indicate malicious activity or system errors. Big data security tools use machine learning algorithms to detect these anomalies in real time.
Patterns refer to recurring sequences that can be observed in data, such as user behavior or network traffic. Identifying these patterns can help organizations predict potential threats and take proactive measures to prevent them. Machine learning algorithms analyze vast amounts of historical data and identify patterns that could indicate an attack or breach.
Predictive Analytics for Threat Detection
By analyzing massive amounts of data, predictive analytics algorithms can identify patterns that indicate potential security threats. This allows organizations to take proactive measures to prevent attacks before they occur.
One key aspect of predictive analytics for threat detection is machine learning. Machine learning algorithms can learn from historical data to identify patterns and anomalies that may indicate a potential attack. As new data is collected, the algorithm continues to learn and improve its ability to detect threats.
Another important aspect of predictive analytics for threat detection is real-time analysis. By analyzing incoming data in real time, organizations can quickly detect and respond to potential threats before they cause significant damage. This requires sophisticated technology that can process large amounts of data quickly and accurately, as well as highly trained analysts who can interpret the results and take appropriate action.
Securing Big Data Infrastructure
Cloud Security Considerations
Cloud security considerations are crucial for any organization looking to adopt these services. One important consideration is data privacy. Organizations must ensure that sensitive information stored in the cloud remains protected and secure from unauthorized access.
Securing Distributed Systems
Maintaining a secure distributed system is crucial to protect sensitive data from unauthorized access. One way of securing distributed systems is by implementing proper authentication and authorization mechanisms. For instance, using strong passwords for user accounts, enforcing multi-factor authentication, and limiting access permissions based on roles can help reduce the risk of improper access.
Another important aspect of securing distributed systems is ensuring data privacy through encryption techniques. Encryption helps in protecting sensitive information while it’s being transmitted between different nodes in the network by scrambling the content in such a way that it can only be decrypted with an authorized key or password. In addition, regular software updates and patching of known vulnerabilities can help keep the system secure against potential threats.
Protecting Data Centers and Networks
Big data security requires sophisticated tools and strategies to manage this volume effectively. Encryption and access controls are essential, but they are not enough on their own. Organizations must also implement intrusion detection systems that can identify and respond to threats quickly.
Big Data Security Tools and Technologies
Encryption and Cryptography
Encryption is the process of converting plain text or data into an unreadable format that can be only read by authorized parties. Cryptography, on the other hand, is the science of writing codes and ciphers to secure communication from third-party interference.
One significant advantage of encryption is that it provides confidentiality for sensitive information such as credit card details, passwords, and personal identification numbers (PINs). Additionally, when combined with other security measures such as firewalls and access controls, encryption can ensure data integrity by preventing unauthorized modifications.
Cryptography helps to ensure data authenticity by confirming the identity of senders and receivers during transmission. This technique uses digital signatures and certificates that confirm that data was sent by an authentic sender. Furthermore, cryptography can provide non-repudiation; this means that once a party commits to sending or receiving any information like agreements or contracts digitally signed with cryptographic techniques cannot deny it later.
Access Control and Authentication Mechanisms
Access control determines who has access to what information within an organization. This is essential because not all employees need access to all data stored in the company’s systems. Authorization mechanisms should be put in place to ensure that only those with the appropriate clearance levels can view, edit, or delete specific files.
Authentication mechanisms are equally crucial because they verify that a user is who they claim to be before granting them access to sensitive data. One of the most common authentication mechanisms used today is two-factor authentication (2FA). With 2FA, users must provide two forms of identification before accessing their accounts, such as a password and a unique code sent via text message or email.
Security Information and Event Management (SIEM) Systems
Security Information and Event Management (SIEM) systems are designed to monitor security events in real time, providing comprehensive visibility into an organization’s security posture. These systems collect and analyze data from various sources, including network traffic, system logs, and security appliances such as firewalls and intrusion detection systems. The insights gained from SIEMs help organizations detect threats quickly, investigate incidents effectively, and implement proactive measures to prevent future attacks.
One of the unique aspects of big data security is the sheer volume of data generated by modern IT infrastructures. Traditional SIEM solutions may struggle to handle this volume without sacrificing performance or accuracy. Fortunately, several vendors offer next-generation SIEM solutions that leverage machine learning algorithms to automate threat detection and response while minimizing false positives.
Blockchain for Data Security
Unlike traditional methods that rely on centralized servers, blockchain distributes and replicates data across multiple nodes, making it nearly impossible to tamper with or corrupt. This decentralized approach not only enhances the security of sensitive data but also ensures its availability and transparency.
In addition to its inherent security features, blockchain technology also facilitates the secure sharing of data among trusted parties by enabling granular access controls and permission management. With this capability, organizations can selectively grant permissions to authorized users while ensuring that unauthorized individuals are blocked from accessing sensitive information.
Big Data Security Best Practices
Implementing a Comprehensive Security Strategy
Organizations must take a multi-faceted approach to security that incorporates both technological solutions and best practices for personnel management. This can include everything from investing in advanced encryption technologies to restricting access to certain types of data on a need-to-know basis.
Training and Awareness Programs
Training and awareness programs are crucial in ensuring the security of big data. The complexity of big data systems can make it difficult for individuals to understand how they function, let alone how to keep them secure. Training programs can help educate employees on best practices for handling sensitive information and detecting potential security threats.
Furthermore, awareness programs can help employees stay informed about emerging risks and the latest tactics used by cybercriminals. This proactive approach to security helps ensure that everyone in an organization is working together to maintain a secure environment for their data.
Regular Vulnerability Assessments and Penetration Testing
Vulnerability assessments help identify potential vulnerabilities in a system, while penetration testing simulates an attack to determine how well a system can withstand an actual breach attempt. These tests help ensure that all potential vulnerabilities are identified and addressed before they can be exploited by attackers.
Conducting regular vulnerability assessments and penetration testing can also help organizations stay compliant with regulatory requirements. Many regulations require organizations to regularly test their systems for vulnerabilities, including the Payment Card Industry Data Security Standard (PCI DSS) and the Health Insurance Portability and Accountability Act (HIPAA).
Incident Response and Recovery Planning
In the context of big data, incidents may take different forms, including cyberattacks, hardware or software failures, natural disasters, and human errors. Organizations with effective incident response plans are better positioned to mitigate the impact of such incidents and minimize disruptions to their operations.
An incident response plan typically involves several stages, including preparation, detection and analysis, containment and eradication, recovery and restoration, and post-incident evaluation. The preparation stage involves identifying potential threats and vulnerabilities in the big data environment and developing a comprehensive plan to respond to them. The detection stage involves using monitoring tools to identify security breaches or anomalies that may indicate an incident has occurred.
The containment stage involves isolating affected systems or networks to prevent further damage or propagation of the incident. The eradication stage involves removing malware or other malicious elements from affected systems. The recovery stage involves restoring normal operations as quickly as possible while minimizing any losses incurred during downtime. The post-incident evaluation stage allows organizations to assess the effectiveness of their incident response plan and identify areas for improvement in future incidents.
Conclusion
It is evident that big data security presents numerous challenges to organizations in different industries. As the volume and complexity of data continue to increase, it is imperative for companies to prioritize cybersecurity measures. The use of encryption, authentication, access controls, and network segmentation can help protect sensitive information and minimize the risk of data breaches.
Moreover, it is important for organizations to have policies and procedures in place for handling data breaches. This involves having a response team that can quickly identify and contain any potential threats while minimizing damage to critical systems. Regular training on cybersecurity best practices should also be provided for employees so as to create a culture of vigilance around the handling of sensitive information.
FAQs (Frequently Asked Questions)
How Is Big Data Security Different From Traditional Data Security?
Big data security is different from traditional data security in several ways. The sheer volume of data in big data systems makes it difficult to monitor and secure. Traditional security measures may not be enough to handle this massive amount of data, which can come from various sources and formats. Big data is often stored in distributed systems, making it more challenging to manage and secure across multiple locations.
Another unique aspect of big data security is the need for real-time processing and analysis. Big data systems generate enormous amounts of information every second, which requires immediate attention to identify potential threats or vulnerabilities. This means that traditional manual analysis techniques are no longer sufficient to detect security issues quickly.
How Can Organizations Protect Sensitive Data In Big Data Environments?
One of the primary methods is by using encryption techniques that ensure that data remains unreadable to unauthorized users. This helps prevent cybercriminals from accessing and stealing sensitive information such as financial records, personal details, and intellectual property.
Another measure is through the use of access control policies that define which users have permission to access specific sets of data. These policies restrict user access based on factors such as their role in the organization or level of clearance. Organizations may also consider implementing multifactor authentication procedures for added security.
Organizations need to monitor their big data networks actively and regularly carry out vulnerability assessments to identify potential weaknesses in their systems. This approach helps detect any suspicious activity early enough before hackers can exploit vulnerabilities, thereby reducing the chances of a successful cyber attack.
How Can Machine Learning Enhance Big Data Security?
Machine learning is an excellent tool that can enhance big data security by detecting and preventing cyber-attacks. It uses algorithms that learn from data patterns to identify potential threats, and it can also automate responses to stop attacks in their tracks. With the increasing amount of data being collected and stored every day, machine learning is becoming more important than ever before.
One way machine learning enhances big data security is through anomaly detection. This technique can detect unusual behavior in real-time, such as unauthorized access attempts or changes to sensitive information. Machine learning algorithms can also analyze large amounts of historical data to identify patterns of attack and predict future threats.
Another area where machine learning plays a significant role in big data security is threat intelligence. By analyzing vast amounts of external threat information, machine learning algorithms can create models that identify specific types of attacks and how they evolve over time. These models can help organizations quickly detect emerging threats and develop countermeasures to prevent them from causing harm.