Defining Data Mining
Data mining is the process of discovering patterns, correlations, and trends within large datasets using statistical and computational methods. It involves extracting useful information from the data and transforming it into an understandable structure for further use. Data mining is used in various fields such as marketing, finance, healthcare, and education to make informed decisions, identify potential opportunities, and mitigate risks. However, data mining also raises concerns about privacy and security as it involves the collection and analysis of personal data.
Significance of Data Mining in Modern Society
Data mining plays a crucial role in modern society as it enables businesses and organizations to make informed decisions based on data-driven insights. For example, in marketing, data mining is used to analyze customer behavior and preferences, which helps businesses to develop targeted marketing strategies and improve customer satisfaction. In finance, data mining is used to identify potential investment opportunities and mitigate risks. In healthcare, data mining is used to analyze patient data and identify potential health risks, which helps healthcare professionals to provide better care and improve patient outcomes.
Understanding the Process of Data Mining
Data mining is a process of extracting useful information and insights from large datasets. It involves various techniques and algorithms to analyze data and identify patterns, relationships, and trends. The process of data mining generally involves the following steps:
- Data collection: The first step is to collect relevant data from various sources such as databases, websites, social media, and other sources.
- Data preprocessing: The collected data is then preprocessed to remove any irrelevant or duplicate data.
Types of Data Mining Techniques
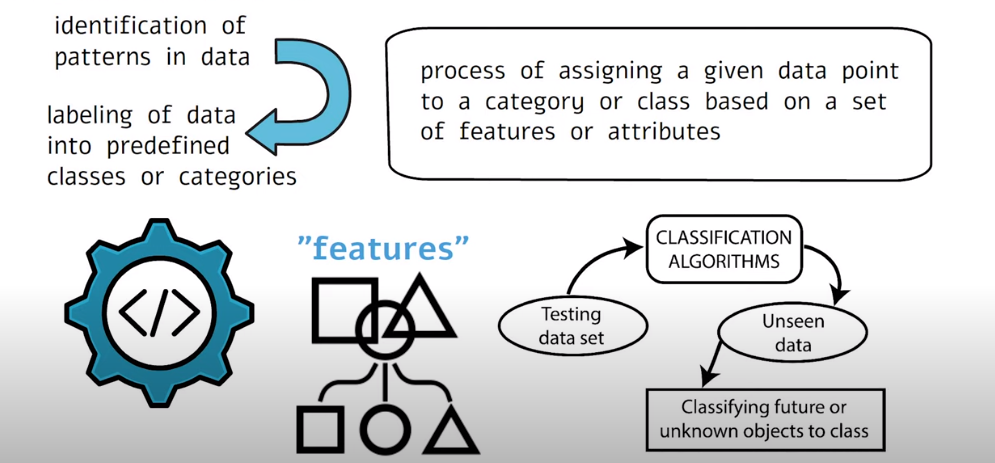
There are several types of data mining techniques used to extract insights from large datasets. Some of the most common ones are:
- Clustering: This technique involves grouping similar data points based on their characteristics or attributes.
- Classification: This technique involves classifying data into predefined categories based on certain characteristics or attributes.
- Regression: This technique involves identifying the relationship between variables and predicting future outcomes based on that relationship.
Data Mining Applications in Various Industries
Data mining has a wide range of applications in various industries, including:
- Finance: Banks and financial institutions use data mining to detect fraudulent activities, identify high-risk customers, and make informed investment decisions.
- Healthcare: Healthcare providers use data mining to analyze patient data and identify patterns that can help in disease diagnosis, treatment planning, and drug development.
- Retail: Retail companies use data mining to analyze customer behavior and preferences, identify trends, and make informed decisions about product pricing and promotions.
The Risks of Data Mining to Personal Privacy
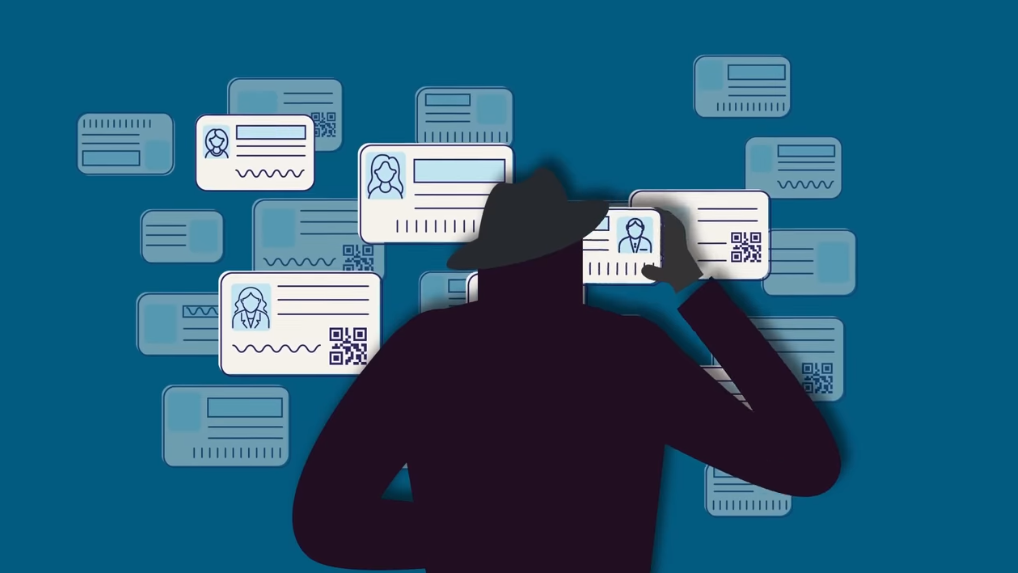
Data mining can pose risks to personal privacy if sensitive information is collected, stored, and used without proper consent or security measures. Some of the potential risks include:
- Identity theft: If personal information such as name, address, and social security number is collected and stored without proper security measures, it can be accessed by hackers and used for identity theft.
- Discrimination: Data mining can lead to discrimination if personal information such as race, gender, and age are used to make employment decisions.
Negative Impact of Data Mining on Society
Data mining can have negative impacts on society if it is used without proper ethical considerations. Here are some potentially negative impacts:
- Privacy invasion: Data mining can invade individuals’ privacy if their personal information is collected and analyzed without their consent.
- Manipulation: Data mining can be used to manipulate individuals’ opinions and decisions by providing them with targeted information that may not be entirely accurate or unbiased.
- Social inequality: Data mining can exacerbate social inequality if it is used to target specific groups.
Privacy Issues with Data Mining
- The Threat of Identity Theft and Fraud
- Invasion of Personal Space
- Biased Decision Making and Discrimination
- Threats to National Security
Techniques for Enhancing Data Privacy
Several techniques can be used to enhance data privacy when conducting data mining activities. Some of these techniques include:1. Anonymization: This involves removing personally identifiable information from data sets before conducting any analysis.
- Encryption: This involves converting data into a coded form that can only be accessed by authorized individuals.
- Access controls: This involves implementing measures to restrict access to data sets to only authorized individuals.
Privacy-Preserving Data Mining Approaches
Privacy-preserving data mining approaches are techniques used to protect sensitive information during data mining activities. These approaches include:
- Differential privacy: This involves adding random noise to data sets to protect the privacy of individuals while still allowing for accurate analysis.
- Secure multi-party computation: This involves multiple parties collaborating to analyze data without revealing sensitive information to each other.
- Homomorphic encryption: This involves encrypting data in a way that allows for computations to be performed on the encrypted data without the need for
Future Trends in Data Mining and Privacy Protection
Several emerging trends in data mining and privacy protection are likely to shape the future of these fields. Some of these trends include:
- Differential privacy: This involves adding random noise to data sets to protect the privacy of individuals while still allowing for accurate analysis.
- Secure multi-party computation: This involves multiple parties collaborating to analyze data without revealing sensitive information to each other.
- Homomorphic encryption: This allows data to be analyzed without being decrypted, providing an additional layer of privacy protection.
- Federated learning: This involves training machine learning models on decentralized data sets, reducing the need for data to be centralized in one location.
- Privacy-preserving machine learning: This involves developing algorithms that can learn from data while still preserving the privacy of individuals in the data set.
Emerging Privacy Risks and Challenges
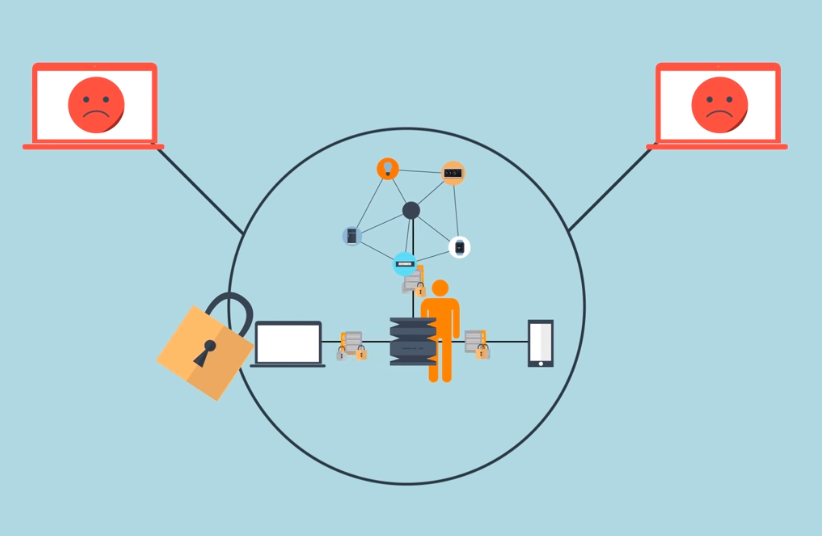
Emerging privacy risks and challenges include the increasing use of facial recognition technology, the proliferation of Internet of Things (IoT) devices that collect sensitive data, and the potential for data breaches and cyber-attacks. Additionally, the privacy implications of artificial intelligence (AI) and automated decision-making are still being explored and debated. Individuals and organizations need to stay informed about these risks and take proactive measures to protect privacy.
Conclusion
In conclusion, privacy is a fundamental right that must be protected in the digital age. As technology continues to advance, individuals and organizations need to be aware of potential privacy risks and take steps to mitigate them. This includes being mindful of the data we share online, using privacy-enhancing tools and technologies, and advocating for stronger privacy protections at the policy level. By working together, we can create a more secure and privacy-respecting digital world.
FAQs
What is Data Mining?
Data mining is the process of analyzing large sets of data to identify patterns, relationships, and insights. It involves using statistical and computational techniques to extract meaningful information from data, which can be used to make informed decisions and predictions. Data mining is commonly used in fields such as marketing, finance, healthcare, and education to uncover hidden patterns and trends in data that can be used to improve business operations and decision-making.
What are the privacy issues associated with Data Mining?
There are several privacy issues associated with data mining. One of the main concerns is the collection and use of personal information without the individual’s knowledge or consent. This can lead to the violation of privacy rights and can potentially be used for unethical purposes such as discrimination or identity theft. Another issue is the accuracy and security of the data being mined. If the data is not properly secured, it can be accessed by unauthorized individuals and used for malicious purposes.
How can organizations protect individuals’ privacy during Data Mining?
Organizations can take several steps to protect individuals’ privacy during data mining. Firstly, they can ensure that they are collecting only the necessary data and that the data is collected with the individual’s consent. They can also implement strict security measures to protect the data from unauthorized access and use. Additionally, organizations can use anonymization techniques to remove personally identifiable information from the data being mined, which can help to protect individuals’ privacy.
What are the legal remedies available for privacy violations in Data Mining?
There are several legal remedies available for privacy violations in data mining. Individuals can file a complaint with the relevant regulatory authorities, such as the Information Commissioner’s Office (ICO) in the UK or the Federal Trade Commission (FTC) in the US. They can also take legal action against the organization for damages caused by the privacy violation.